DATA SHARING
Sharing your data in an effective and efficient has two important aspects:
Self-service publication: Provide your teams, departments or partners a central location to publish data in a very easy way, allowing them to offer a wealth of consumption methods and powerful data storytelling capabilities.
Self-service consumption: Provide end-users the ability to easily find data, background information and access to data. Enable them to find, select and consume the data easily, according to their specific needs. Let them easily understand your data tales and messages. Provide your end-users the ability to visually analyze your data and enable them to create their own data stories
In this way you can unlock significant value from your data, drive innovation, and contribute to collective knowledge.
Self-service Data Publication
Self-service data publication enables internal teams or external partners to independently publish and share their data assets, typically through a data portal or data marketplace, without needing IT intervention. It empowers individuals to make data accessible for broader use, fostering a culture of data collaboration and democratization across the organization.
Important for self-service data publication:
User-friendly user experience: Very simple and attractive interfaces, often within a data catalog or data marketplace, allow data owners to manage and publish data without technical expertise.
Metadata and documentation: Data publishers can add descriptions, data lineage, and other relevant metadata to provide context and ensure that end-users understand the data and its limitations.
Access controls and permissions: Data owner should be able to define who can see, access or use specific datasets, ensuring security and compliance with organizational and regulatory standards.
Offering standard consumption models: By publishing dataset publishers provide standard ways to consume the data, browsing, downloading in different formats, using graphs or viewing it on a map, or API’s for custom usage or application integration.
A data publisher should not need to do anything special to offer this.Ability to create data stories: A data publisher needs powerful tools to be able create data stories from a dataset using narratives and visualizations. This in order to assure effective communication of intended messages and insights.
Usage monitoring: Data publishers should be able to see and monitor how uses their data assets and how it is used.
Self-service Data Consumption
For effective self-service data consumption you need specific features and capabilities that focus on enabling you to easily find, access, understand, analyze, and derive insights from data. As a data-consumer you require a user-friendly, versatile experience that supports a wide range of use cases, from quick data exploration to advanced analytics or advanced capabilities for integration of data assets in your own applications or business processes.
Important for self-service data consumption:
User-friendly data discovery: Advanced and intelligent search capabilities, such as keyword, filter-based or semantic search help users locate relevant datasets quickly.
Easy-to-use visualization tools: Simple, built-in visualization options (charts, graphs, maps) that allow me to quickly view, analyze and interpret the data without needing external tools.
Metadata and context: Relevant metadata and information to provide context, so I can understand the data, its meaning, significance, sources, lineage, relationships and limitations.
Data access and integration flexibility: Different ways to select and retrieve the data
File download options: Multiple format options (e.g., CSV, JSON, Excel, Parque, ) for data downloads to make it easy to access data in a preferred format for further analysis.
APIs for data consumption: APIs to facilitate programmatic data access, allowing me to seamlessly pull data into other applications, analytics tools, or dashboards.
Performance and scalability: A reliable performance when data volumes are big and usage is wide, enabling me to consistently access the data without delay or downtime,
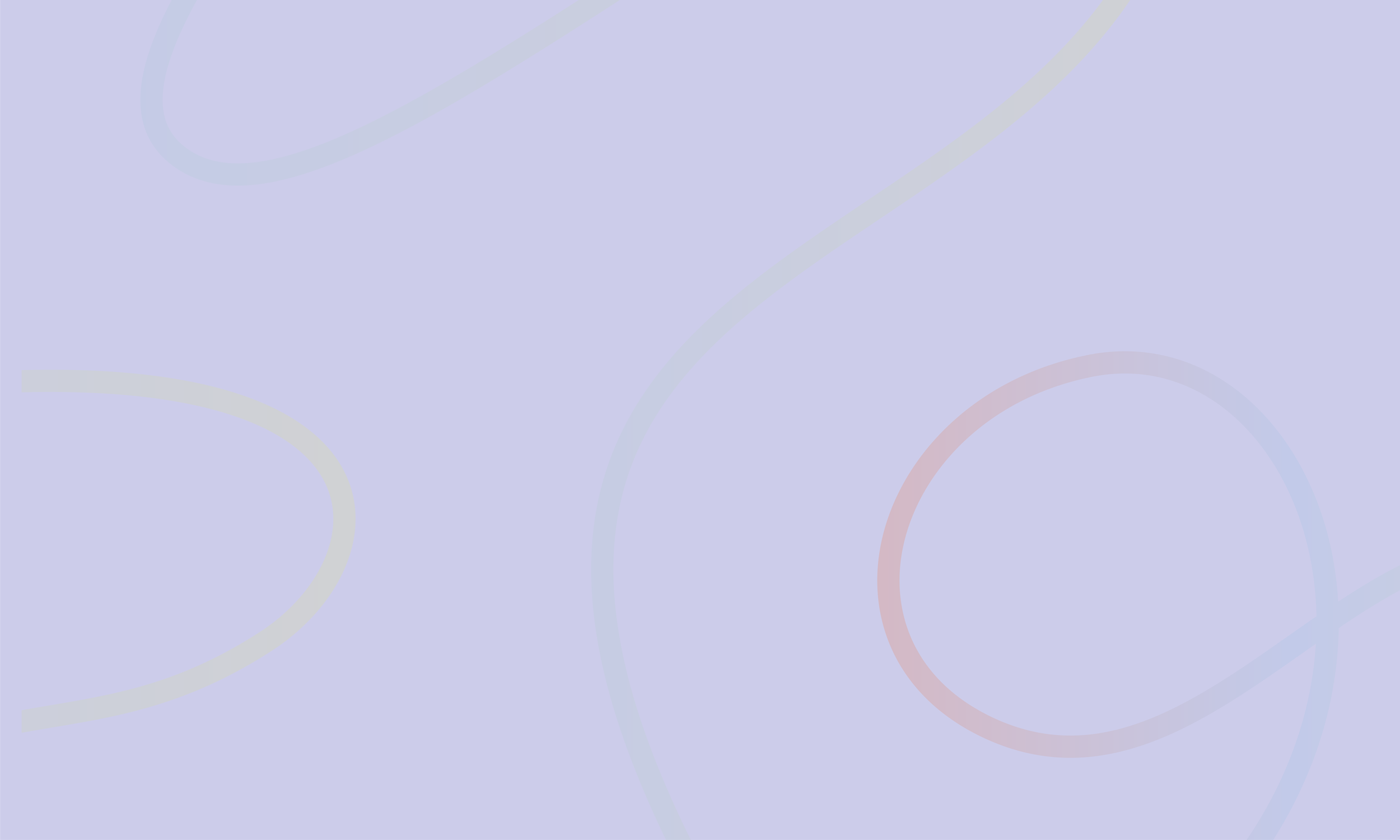
Data Story Telling
Data storytelling combines data, visualizations, and narrative to convey insights effectively. It helps audiences understand complex data through relatable stories. By doing this in a business friendly way, it engages audiences, drives decision-making and facilitates better communication of findings.
Data storytelling is the practice of combining data analysis with narrative techniques to communicate insights in a compelling, relatable, and easily understandable way. This approach not only involves presenting data visually but also integrating context, emotion, and a storyline that guides the audience through complex information toward a clear understanding and takeaway.
Key components of data storytelling:
Data: Raw figures, metrics, and statistics that provide the factual basis for the story.
Narrative: A storyline or structure that connects the data points, giving them context and explaining why they matter.
Visuals: Graphs, charts, or infographics that help convey information visually, making complex data more accessible and engaging.
Why data storytelling is important
Engagement: People are naturally more engaged with stories than raw data, as stories are easier to understand and remember. This approach makes data more accessible, especially for non-technical audiences.
Improved decision-making: Data storytelling helps decision-makers see beyond the numbers by connecting data to real-world outcomes. It can highlight trends, patterns, and potential implications, allowing stakeholders to make informed choices.
Emotional connection: Data on its own is impersonal, but storytelling can add a layer of empathy or urgency that resonates with the audience. This can drive action, especially when the data pertains to issues that impact people or communities.
Clarity and focus: Storytelling helps to distill complex data into a focused message, allowing the audience to concentrate on the key insights without being overwhelmed by extraneous details.
Persuasion: A well-told data story can be persuasive, showing both evidence and context to support a specific viewpoint or recommendation. By framing data within a narrative, analysts can guide audiences toward understanding the significance and urgency of the insights.